Solving the Counterparty Default Scenario Problem
This article introduces Credit Risk Cascades, a new model that forecasts the probability of default of financial institutions under compound scenarios. The model seamlessly integrates macroeconomic, counterparty, and systemic risk projections. This article illustrates the use of the Credit Risk Cascades tool via a case study for US financial firms under the CCAR 2016 baseline and severely adverse macroeconomic scenarios.
Introduction
Thinking in terms of compound scenarios, which are comprised of macroeconomic, counterparty, and systemic risk components, represents the natural next step of stress testing. For eight bank holding companies, the Fed now requires layering a counterparty default scenario onto standard CCAR exercises, and has recently suggested that next-generation stress tests could feature shocks to bank interconnectedness. In fact, as reported in American Banker, Fed governor Daniel K. Tarullo has recently gone on record stating that he "...envisions the stress-testing process moving beyond just examining the performance of individual banks' capital levels under stress and also include the interconnection of institutions under stress as well."1 When forecasting probability of default (PD) for such purposes, banks require a model that seamlessly integrates macroeconomic, counterparty, and systemic risk projections. In fact, some large banks that conduct sophisticated "war game" exercises as part of their risk management activities already think in these terms, but lack a model that allows a fully coherent evaluation of such shocks together. The banking industry needs a streamlined quantitative tool to facilitate stress testing under compound scenarios.
In this article, I introduce a new model – Credit Risk Cascades (CRC) – that forecasts PDs of financial institutions under compound scenarios involving economic, counterparty, and systemic risk components. The CRC model incorporates these three main effects:
- Direct dependence of PD forecasts on the economic scenario
- Credit risk spillovers via network linkages
- A user-specified path for financial sector interconnectedness
This is accomplished with the following techniques:2
- Using Moody's Stressed EDF to obtain EDF paths under a user-selected economic scenario
- Using a dynamic network model to obtain PD forecasts under the counterparty shock relative to an appropriate no-shock baseline
- Adjusting the Stressed EDFs to take into account the network model results
Here, I illustrate the use of the Credit Risk Cascades tool via a case study for US financial firms under the CCAR 2016 baseline and severely adverse macroeconomic scenarios. EDF forecasts under these scenarios, obtained from Stressed EDF, are modified in response to the default of a small set of counterparties in a way that allows credit risk shocks to propagate more strongly when bank interconnectedness is high. After discussing other regulatory applications of CRC and providing a brief methodology overview, the case study illustrates the effects that different counterparty and systemic risk events could have for a select group of major US banks under alternative paths for the macroeconomy.
Regulatory Context: Other Applications of CRC
While the counterparty default scenario that forms the focus of this article is a key regulatory application of CRC, it is not the only one. Two other regulatory applications include single counterparty credit limits (SCCL) and compliance issues related to the "living will" portion of Dodd-Frank.
Related to the first point, the Fed has recently proposed an update to the rules governing SCCLs for US bank holding companies and foreign banking organizations with at least $50 billion in total consolidated assets. As part of the proposed rules, whenever a bank holding company's net credit exposure to an unaffiliated counterparty exceeds 5% of the bank's eligible capital, the bank must determine whether the counterparty is economically interdependent with any of the bank's other unaffiliated counterparties.3 The analysis required to determine such a relationship turns directly upon whether the financial distress of one counterparty is likely to impair the ability of other bank counterparties to make good on their liabilities to the bank. As the case study of this article illustrates, CRC quantifies precisely the extent to which the distress of one counterparty will transfer to another counterparty of interest under a variety of economic scenarios.
Regarding the living wills required of major US banks under Dodd-Frank, the Federal Reserve and Federal Deposit Insurance Corporation (FDIC) recently said that the resolution plans submitted in 2015 by five of the largest US banks failed to pass muster and must be rewritten by the banks prior to October 1, 2016.4 Among the faults found in the living wills, regulators cited the failure to fully address the material financial interconnections between banks and broker-dealers that would be relevant when winding down trading portfolios. Network methodologies such as the one behind CRC are fit-for-purpose for quantifying the strength and direction of such interconnections under economic stress.
Methodology Overview
The CRC model builds on Systemic Risk Monitor5 for network model estimates, and on Stressed EDF6 for projections of EDFs conditioned only on the macroeconomic scenario. CRC combines the information in the network model and Stressed EDF to arrive at the CRC-EDF forecast paths for financial institution PDs under the compound scenario, which includes the macroeconomic scenario as well as the systemic risk path and counterparty shocks specified by the user. All of the aforementioned methodologies employ the most recent iteration of CreditEdge, the EDF9 measure, as the core input. The construction of this newest iteration of the EDF metric of default probability and differences with the previous version are documented in detail in Chen et al. (2015).
The financial network I use to drive this application of CRC is the Global Megabanks network, which consists of all publicly traded financial institutions whose book assets exceed $100 billion in value. Historical estimates of this network's structure and associated systemic risk measures are provided in Hughes and Malone (2015), and were updated for this study using the most recent available CreditEdge data. The Global Megabanks network is the natural peer group of global CCAR-sized institutions in general and the institutions subject to the counterparty default scenario in particular. Systemic Risk Monitor estimates the extent and strength of Granger causal connections between the EDF series of all distinct pairs of financial institutions in the network, and this information is passed to CRC for forecasting.
Case Study
The base date for our compound counterparty default scenario is February 2016. For illustrative purposes, I consider shocks to two counterparties: Fifth Third Bancorp and US Bancorp. Both of these firms are subject to CCAR stress tests but are not required to do the counterparty default scenario. I adopt a 27-month time horizon and forecast default probabilities for each month during that period for two firms that are required to do counterparty default scenarios under CCAR: Bank of New York Mellon and JP Morgan Chase.
As stated in the previous section, I measure PDs using the Moody's CreditEdge EDF metric, which provides an estimate of the 1-year ahead probability of default. CRC and Stressed EDF both forecast EDFs under scenarios, and I will refer to these forecasts as CRC-EDFs and SEDFs, respectively. CRC-EDFs are compound scenario forecasts, whereas SEDFs are forecasts conditional on only the macroeconomic component of the scenario. For the same underlying Fed macro scenario, their difference can be attributed to counterparty shocks and the effect of changes in financial sector interconnectedness.
In CRC, counterparty shocks take the form of perfect-foresight PD paths. In other words, the user specifies the time series that a given bank's PD will follow over the course of the forecast horizon, and the CRC algorithm solves for the PDs of other banks endogenously using model averaging to construct forecasts. In this case study, I assume that from month 0 (February 2016) until month 27 (May 2018) of the forecast horizon, the PDs of both Fifth Third Bancorp and US Bancorp follow the path taken by the EDF of Lehman Brothers during the 28 -month period from January 2008 to April 2010. During this period, Lehman Brothers' EDF reached the maximum value for financial firms of 35% for the first time in September 2008, or month 8, and remained there for the rest of the time horizon.
Compound scenarios are closed by specifying macroeconomic and systemic risk components. The macro scenario choice set for our purposes consists of the 2016 Fed baseline scenario and the 2016 Fed severely adverse scenario. For the systemic risk scenario choice set, I consider two alternatives: network stasis and network stress. Under network stasis, it is assumed that the DGC.plus interconnectedness measure for the Global Megabanks network will remain at its February 2016 value over the scenario time horizon. Under network stress, in contrast, the DGC.plus measure is assumed to follow the path it took during the turbulent period from January 2008 to April 2010, to coincide with the same period from which the Lehman Brothers EDF values are sourced for the counterparty shock. The time paths of the DGC.plus measure under network stasis and stress are depicted in Figure 1.
Figure 1. Interconnectedness measure under network stasis and network stress
Source: Moody's Analytics
The results are organized into four figures of four plots each. Each individual plot displays the CRC-EDF and SEDF of either Bank of New York Mellon or JP Morgan Chase under a specific compound scenario. Each of the four figures corresponds to a choice of macroeconomic scenario (Fed baseline or Fed severely adverse) and a choice of systemic risk scenario (network stasis or network stress). For a given figure, each of four plots corresponds to one of the four choices of shocked counterparty bank (Fifth Third Bancorp or US Bancorp) and bank whose shocked CRC-EDFs and SEDFs are displayed (Bank of New York Mellon or JP Morgan Chase).
Results
Results are displayed in Figures 2 through 5. Figure 2 shows CRC-EDF9 and SEDF9 paths under the Fed baseline macro scenario and network stasis. Figure 3 shows these series under the Fed severely adverse macro scenario and network stasis. Figure 4 shows results for the Fed baseline macro scenario and network stress, and Figure 5 shows results for the Fed severely adverse macro scenario and network stress.
As seen in the left column of Figure 2, a shock to Fifth Third Bancorp has a small negative effect on the CRC-EDF of BNY Mellon relative to its Stressed EDF. The Fifth Third Bancorp shock produces virtually no effect on the CRC-EDF forecast relative to the Stressed EDF for JP Morgan. In contrast to the case of the Fifth Third Bancorp shock, the distress and subsequent default of US Bancorp would nontrivially raise the default probabilities of both JP Morgan Chase and BNY Mellon, even under network stasis. This can be seen in the right column of Figure 2.
Banks could incorporate private information on their own direct counterparty exposures to further increase the realism of results.
As is evident in the right-hand columns of all four figures, BNY Mellon's EDF would be impacted more than that of JP Morgan Chase in response to a US Bancorp default. These findings illustrate the role that Credit Risk Cascades might play in counterparty selection in the context of bank stress testing.
Figure 2. CRC-EDF9 and SEDF9 paths under Fed baseline and network stasis
Source: Moody's Analytics
It is useful to compare Figures 2 and 4. In Figure 4, the small deviations from Stressed EDF under the Fed baseline scenario are magnified when we increase the level of interconnectedness in the financial network, and the net effect of a Fifth Third Bancorp shock on JP Morgan Chase's EDF forecast turns positive under network stress. This result illustrates the importance of risk transmission via the intermediate counterparties connecting the two banks in the network.
Figure 3. CRC-EDF9 and SEDF9 paths under Fed severely adverse and network stasis
Source: Moody's Analytics
Additionally, a comparison of the right-hand columns of Figures 2 and 4, as well as of Figures 3 and 5, shows that a heightened level of interconnectedness in the financial network would exacerbate the effect of the US Bancorp counterparty shock on both banks. In general, the counterparty-systemic interactions impact BNY Mellon and JP Morgan Chase differently depending on which counterparty we shock. These results illustrate the potential for counterparty shocks to be more damaging when the strength and prevalence of credit risk spillovers in the financial network increase, as is the case during periods of market stress.
Figure 3. CRC-EDF9 and SEDF9 paths under Fed baseline and network stress
Source: Moody's Analytics
We can gain specific insight on the relative contributions of the three components of the compound scenario to the resulting CRC-EDF of a bank by decomposing the PD forecast on a specific date. Let us take as an example the case of BNY Mellon in February 2017, one year after the scenario begins.
In February 2017, BNY Mellon's CRC-EDF under the compound scenario involving (a) the Fed severely adverse macroeconomic scenario, (b) a counterparty shock to US Bancorp, and (c) network stress – located in the upper right corner of Figure 5 – is approximately 4.2 percent. This compares to the Stressed EDF on the same date under the Fed baseline scenario of just under 0.5 percent, as shown for example in the upper right corner of Figure 2. Let's decompose this difference of 3.7 percentage points.
Figure 5. CRC-EDF9 and SEDF9 paths under Fed severely adverse and network stress
Source: Moody's Analytics
Compared to the SEDF under the Fed baseline, adding macroeconomic stress alone accounts for an increase of 2.2 percentage points in the EDF. This gets us to a SEDF under the Fed severely adverse scenario of 0.5 + 2.2 = 2.7%, as shown in the upper right-hand corner of Figure 3. From there, the US Bancorp counterparty shock accounts for an additional 1 percentage point increase in the EDF, taking us to 3.7 percent under the scenario involving the Fed severely adverse macro component, the US Bancorp counterparty shock, and network stasis. This result can be seen by inspecting the CRC line in the upper right-hand corner of Figure 3. Layering on financial network stress accounts for the remaining 0.5 percentage point increase, to bring us to the CRC-EDF of 4.2 percent under the most stressful compound scenario, shown in Figure 5. The relative magnitudes of these contributions to the CRC-EDF accord with intuition about their relative importance in most situations.
CRC does not use information on direct counterparty exposures on banks' books. Rather, it uses information from the last five years of history to estimate the presence and strength of spillovers between the PDs of all pairs of banks in the financial network. This produces real-time, market-based conditional forecasts of financial institutions' PDs under macroeconomic, systemic, and counterparty stress.
Without a doubt, banks could incorporate private information on their own direct counterparty exposures to further increase the realism of results, such as by shocking their assets directly in the face of a projected counterparty default. The shocked bank assets would flow through the structural formula that drives the bank EDF, and this direct impact could be overlaid on the results provided by CRC. Alternatively, banks could use their own PD series to calibrate the model instead of using CreditEdge EDFs, so long as their PD series are available with sufficient frequency (monthly) and history (at least five years is recommended).
An important advantage of CRC is that it allows risk managers to view counterparty default as the culmination of a process of deteriorating credit quality. Such deterioration manifests itself in the form of persistent increases in the probability of default over time, and CRC allows the user to take a granular view of how the PDs of different counterparties might evolve over the forecast horizon as their projected default events draw near.
Finally, while banks know who their own counterparties are and their exposure to them, they know comparatively little about their counterparties' counterparties. This lack of knowledge can be most dangerous precisely when it matters most: in times of market stress. Banks take it for granted that the default of a direct counterparty will affect them, but often gloss over the potential for that default (or default by a third party) to take down a second of their important counterparties. CRC uses network models and model averaging techniques to create conditional forecasts of financial firm PDs. In a backtest using data from the financial crisis, forecasts conditional on appropriately selected compound scenarios proved superior to PD forecasts conditional on macroeconomic stress alone (Malone, 2015).
Conclusion
This article introduces a new solution, Credit Risk Cascades, for the Fed's counterparty default scenario requirement in the latest installment of CCAR. The CRC model allows users to evaluate the impact on firm default probabilities of compound scenarios. Such scenarios involve a macroeconomic scenario component, a systemic risk component, and a counterparty distress or default scenario for one or multiple counterparties of interest. CRC integrates the effects of these three types of shocks together seamlessly to forecast the PDs of all firms in a user-specified financial network. While banks know who their own counterparties are and their exposure to them, they know comparatively little about their counterparties’ counterparties. This lack of knowledge can be most dangerous precisely when it matters most: in times of market stress.
While banks know who their own counterparties are and their exposure to them, they know comparatively little about their counterparties’ counterparties. This lack of knowledge can be most dangerous precisely when it matters most: in times of market stress.
Using data on default probabilities from Moody's CreditEdge, bespoke networks can be constructed and shocked for publicly traded financial institutions around the world. CRC can be used to select counterparties for default way can help banks construct more effective counterparty default scenarios. The case study presented here, based on the network of Global Megabanks with at least $100 billion in assets, shows that both appropriate counterparty selection and a rise in financial sector interconnectedness can cause material changes in projected credit quality for banks subject to CCAR.
Finally, the regulatory applications of CRC are not limited to the counterparty default scenario alone. Single counterparty credit limit (SCCL) regulations and the need to better take into account interconnectedness when devising resolution plans, or "living wills," also provide highly relevant applications for this network-based solutio
Footnotes
1 Heltman, 2015.
2 The full documentation and validation of Credit Risk Cascades can be found in Malone (2015).
3 An unaffiliated counterparty is generally understood to be one that the bank does not control, is not controlled by the bank, and is not under common control with the bank that is the subject of SCCL regulation.
4 Hamilton & Dexheimer, 2016.
5 This is documented in Hughes & Malone (2015).
6 This is documented in Ferry, Hughes, & Ding (2012).
Sources
Ferry, Danielle, Anthony Hughes, and Min Ding, Stressed EDF Credit Measures for North America, Moody’s Analytics whitepaper, May 2012.
Malone, Samuel W., Credit Risk Cascades: Forecasting PDs under compound scenarios, Moody's Analytics whitepaper, November 2015.
Hamilton, Jesse, and Elizabeth Dexheimer, Five Big Banks’ Living Wills are Rejected by U.S. Regulators, Bloomberg News, April 13, 2016.
Heltman, John, "How the Fed Might Change Stress Tests in Version 2.0", The American Banker, December 28, 2015.
Hughes, Anthony and Samuel W. Malone, Systemic Risk Monitor 1.0: A Network Approach, Moody's Analytics whitepaper, June 2015.
Chen, Nan, Houman Dehghan, Min Ding, Jian Du, Douglas Dwyer, James Edwards, Danielle Ferry, Pooya Nazeran, Zhao Sun, Jing Zhang, and Sue Zhang, EDF9: Introduction and Overview, Moody’s Analytics whitepaper, June 2015.
Featured Experts
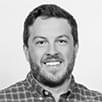
James Partridge
Credit analytics expert helping clients understand, develop, and implement credit models for origination, monitoring, and regulatory reporting.

Avinash Arun
Creates credit assessment and origination strategies and supports commercial loan origination and risk management objectives.
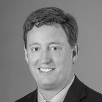
John Baer
Credit risk expert; data analytics and technology process consultant; former M&A advisor for private equity and corporate clients
As Published In:
Analyzes IFRS 9, delves into its effects on future impairment calculations, and provides recommendations on how financial institutions can implement and leverage forward-looking credit loss models.
Previous Article
Are Deposits Safe Under Negative Interest Rates?Related Articles
Credit Risk and Bond Spreads
This article undertakes a thorough exploration of the dynamic relationships linking credit risk and bond spreads. Credit metrics help forecast absolute spreads and relative bond returns.
Moody's Analytics EDF-Based Bond Valuation Model Version 2.0
This paper gives an overview of the Moody’s Analytics model of bonds' Fair Value Spread and Alpha Factor.
Evaluando el Riesgo de Crédito bajo el Coronavirus (COVID-19)
En este seminario web, utilizaremos las métricas EDF de Moody’s Analytics para evaluar el impacto que COVID-19 ha tenido hasta ahora en el riesgo de crédito.
The coronavirus (COVID-19) pandemic: Assessing the impact on corporate credit risk
As COVID-19 spreads globally, fear and uncertainty are rising, roiling financial markets and pushing the global economy towards recession. This report uses Moody’s Analytics CreditEdge™ public-firm EDF™ (Expected Default Frequency) metrics to assess the impact that the coronavirus has had so far on credit risk.
The COVID-19 Pandemic: Assessing the Impact on Corporate Credit Risk
In this webinar, we will use Moody’s Analytics EDF metrics to assess the impact COVID-19 has had so far on corporate credit risk.
Dealing With Fallen Angel Risk
Highly Commended for Best Fixed Income Paper in the 2019 Savvy Investor Awards, this paper tests the early warning power of the CreditEdge Deterioration Probability (DP) metric for Fallen Angel downgrades.
Moody's Analytics Webinar: Environmental, Social & Governance and Credit Risk Ideas for Fixed Income Investors
Fixed income and equity investors face growing pressure to measure and monitor the ESG risk of their portfolios.
Moody's Analytics Webinar: Topics@CreditEdge – Dealing With Fallen Angel Risk
Fallen Angel risk results from the possibility and price impact of bond downgrades from investment grade (IG) into high yield (HY).
Moody's Analytics Webinar: Topics@CreditEdge Navigating Choppy Markets - Focus on Asia
Since the Asia crisis, most countries in Asia have displayed a longer term secular trend of falling default risk.
Topics@CreditEdge Webinar – Navigating Choppy Markets: Safety-First Equity Strategies Based on Credit Risk Signals
Join us as our experts discuss the use of credit risk measures in evaluating firms to determine tendencies in performance in comparison to their peers in the S&P 500 universe.