Leveraging Basel III Compliance Implementations
This article examines how regulatory compliance initiatives worldwide have shaped current risk management systems and practices. It then covers the challenges and benefits of funds transfer pricing practices, profitability analysis, and stress testing-based governance practices.
Basel III compliance implementations have significant implications for risk management practices across the banking industry, and bring data management, risk model, and infrastructure challenges.
Basel requirements have shaped typical risk management systems and practices
Across most financial institutions worldwide, front office transaction data is processed within separate risk management data flows. In a nutshell, there are three main uses of transaction data:
- Market risk practices look into short-term risks relevant to the trading book
- ALM/finance practices provide long-term banking risk and profitability analytics
- Credit risk measures address long-term default risk and solvability of the portfolio
In terms of data flow organization, the separate focus on different risk measurements, various time horizons, and risk factors in the simulations have led to a consistent view across the financial industry. Overall, the risk management organization, roles, and systems are typically shaped according to the three main data flow silos: Market Risk Office, Asset and Liability Committee (ALCO), and Credit Risk Office (see Figure 1).
Within the structure of governance and risk management systems, each silo relies on its own set of models relevant to a specific set of front office transactions. Additionally, data volumes differ significantly in the trading and banking books, leading to independent processes whose reporting updates range from intraday for market risk to monthly for governance and forecasts practices. Overall, this organization of tasks has emerged from successive waves of business and regulatory implementations and is now generally accepted in the industry as common sense.
The previous Basel II regulatory wave reinforced these silos, establishing a new emphasis on credit risk practices and raising the complexity of data requirements. It also sparked group-wide consolidation of granular-level data, while allowing other silos, such as market risk and ALM, to remain unchanged.
Along with the enhancement of credit assessment models, credit risk-weighted asset (RWA) requirements have also spurred the evolution of risk management infrastructures. Detailed data requirements at the transaction, collateral, counterparty, and guarantee levels have increased the need for data warehouses, which collect and centralize data from front office transactional systems, and validate and improve data quality controls. The use of data warehouses has often remained limited in scope as daily data flow frequency and daily T+1 processes, required in the market risk and ALM silos, have prevented their application across all silos. Measurements leveraging trading book data typically require a direct feed from treasury systems, rather than collecting it from a data warehouse.
Figure 1. Typical risk management data flow silos in financial institutions
Source: Moody's Analytics
Overall, this creates the need to build a new data flow that assembles the components on a daily basis, as illustrated in Figure 3.
The impact of Basel III liquidity monitoring requirements
With new Basel III requirements, come new challenges to the dataflow structure. Relying on both cash flow projections and credit risk weighting and classification, the Basel III driven contingent liquidity planning framework requires, within a daily data flow, the merging of ALM models with credit behaviors. Pillar II liquidity monitoring requirements reinforce the need to revisit cash flow models to account for asset classes and forecast credit transitions.
Creating relevant models for credit and cash flow behavior over a 30-day time horizon is complicated by technical data limitations that are often observed on the credit model side. Indeed, whereas Pillar I liquidity compliance leverages public credit ratings, such credit assessments result in only scarce historical time-series, which prevents banks from adequately refining credit transitions and calibrating liquidity models.
A typical requirement, illustrated by the European Banking Authority (EBA) publication, Additional Liquidity Monitoring Metrics, consists of providing a view of an institution’s internal liquidity behavioral forecasts, projected on the regulatory Liquidity Coverage Ratio (LCR) classification. This particular report requires an improved set of explanatory factors for the cash flow models in the simulation, accounting for a new segmentation of internal behavioral models, according to counterparty classifications and rating behaviors.
Including credit transitions into such models provides its own challenges. Similar to approaches used in credit economic capital simulations, monitoring LCR over a 30-day horizon demands an accurate and forward-looking understanding of credit transitions. This provides an accurate measurement of the high-quality liquid assets forecast, helping perform informed asset selections that are eligible in the numerator of the LCR. It also allows banks to properly anticipate the evolution of the inflow and outflow performance on the LCR denominator.
Creating relevant models for credit and cash flow behavior over a 30-day time horizon is complicated by technical data limitations that are often observed on the credit model side. Indeed, whereas Pillar I liquidity compliance leverages public credit ratings – providing a public and auditable source of data consistent with financial statements – such credit assessments result in only scarce historical time-series with at most one observation point every quarter. This lack of granular historical credit data prevents banks from adequately refining credit transitions and calibrating liquidity models.
Figure 2. Creation of data warehouses often triggered by Basel II initiatives
Source: Moody's Analytics
For internal liquidity contingency planning purposes, risk managers now tend to leverage equity prices and sovereign credit default swap (CDS) spreads-based ratings. Not only do these approaches reflect a forward-looking appreciation of credit by market analysts, but they also provide a suitable set of historical time-series to run against historical cash flows and balances observations, resulting in fine-grained and responsive calibration of behavioral models.
Moody’s Analytics currently sees the emergence of this type of usage among its clients, for cross risk models calibration and specifically short-term liquidity inflows and outflows monitoring.
With regard to the Liquidity Coverage Ratio, monitoring sovereign bonds that are eligible in the numerator also demands a forward-looking and responsive assessment of credit, which CDS-implied EDFs can provide.
Figure 3. Basel III liquidity requirements combine cash flow and credit analysis
Source: Moody's Analytics
FTP and profitability analysis
With regard to funds transfer pricing (FTP), Basel III liquidity requirements now introduce a review of loan and commitment prices, accounting for new contingent liquidity spreads and an associated profitability analysis. To achieve proper profitability measurements, FTP models will now have to rely on data and cash flows that originate from the liquidity contingency planning silo. As a logical step, ALM managers are now looking to leverage this FTP practice. This makes the dataflow – newly built for contingent liquidity planning – a good starting point for balance sheet forecasts, pro forma FTP models, and profitability analysis.
In doing so, Basel III liquidity requirements create the opportunity for financial institutions to generate a consistent view of analytics across credit, liquidity, interest rate risk, and profitability. This revamped data flow, created for compliance purposes, is worth the investment. It provides a solid foundation for upcoming regulatory stress testing automation requirements and modern, macroeconomic scenario-driven governance practices.
Recent governance practices, proposed by US and European stress testing regulators, can leverage the results from the ALM-Credit- Liquidity silos as a strong starting point. This framework allows banks to perform appropriate measurements on a number of key aspects, such as the Asset Quality Review (AQR) and data harmonization, Internal Capital and Liquidity Adequacy Assessment, risk appetite definition, FTP and limit policies settings, and profitability planning and forecasting.
In this approach, macroeconomic models are applied to key portfolio risk and performance indicator forecasts within a lightweight simulation framework, providing fully auditable measurements. By allowing banks to operate a meaningful number of governance assumptions within a short turnaround time, this framework enables a fruitful dialogue between the governance team and senior management, based on macroeconomic forecasts and governance hypotheses. Implemented as an autonomous computational layer, such processes can be performed independently from pre-existing risk infrastructures, creating little-to-no impact on existing risk management production environments, providing the institution with a cost efficient implementation for stress testing automation workflows.
Figure 4. Evolution of behavioral cash flow models to account for counterparty credit
Source: Moody's Analytics
Over the years, regulatory compliance implementations have promoted a gradual and consistent evolution of risk practices and infrastructures within financial institutions. Along with enhancing banks’ solvability, Basel II helped financial institutions improve their data management capabilities. Adopting a strategic approach to implementing Basel III liquidity compliance and monitoring can provide significant short-term returns on investment – differentiating an institution’s ability to leverage, in a cost efficient way, a unified view of data, risk profiles, and behaviors for improved balance sheet forecasts, profitability analysis, and stress testing-based governance practices.
Figure 5. Comparison of financial statement-based ratings and market prices-based ratings, providing fine-grained historical time-series
Source: Moody's Analytics
Figure 6. Finance and ALCO leverage liquidity data and FTP models enrichment for profitability forecasts and analysis
Source: Moody's Analytics
Figure 7. Deployment model for stress testing automation, leveraging outputs from existing data flows within a streamlined and efficient simulation and governance framework
Source: Moody's Analytics
Featured Experts
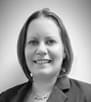
Karen Moss
Senior practitioner in asset and liability management (ALM) and liquidity risk who assists banking clients in advancing their treasury and balance sheet management objectives
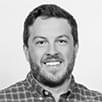
James Partridge
Credit analytics expert helping clients understand, develop, and implement credit models for origination, monitoring, and regulatory reporting.
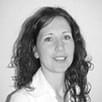
María Cañamero
Skilled market researcher; growth strategist; successful go-to-market campaign developer
As Published In:
Details how global risk managers can comply with new regulations, better manage risk, and meet business and industry demands.
Previous Article
The Benefits of an Integrated Risk Management Framework within BanksRelated Articles
Reducing Volatility in IFRS9 Provisions & Earnings, Through Governance and Credit Decision
As preliminary IFRS9 results are being released, many institutions have concerns about variations in point-in-time credit assessment and forward-looking credit forecasts. These measurements are responsive to the economic environment, and highly dependent on changes in an institution’s macroeconomic outlook.
Anticipating and Benchmarking Variance in IFRS 9 Expected Credit Losses
Many financial institutions are designing their model overlay with a view to manage macroeconomic forecast uncertainty and model risks. For this purpose, aside from the expected credit losses, risk management teams can provide the finance department with more measurements to anticipate variability and uncertainty levels around expected credit losses. This document discusses risk measurements that can be leveraged to achieve these objectives.
Webinar with the Asian Banker: Managing IFRS 9 expected credit losses and forecast
For IFRS 9 impairment calculations, point-in-time forward-looking credit assessments are prone to be responsive to the economic environment and the periodic revision of the economic outlook. Therefore, the management of provision variances over time is a particular area of focus.
Managing IFRS 9 expected credit losses variance and forecast uncertainty
As financial institutions are currently focusing on the execution of their IFRS 9 program and solution integration, risk and finance teams are working together to anticipate their effect on the financial reports. Especially, on the impairment modeling side, point-in-time forward-looking credit assessments are prone to be more responsive to the surrounding economic environment than the through-the-cycle measurements in practice so far. As institutions are anticipating some variability of provisions levels in relation to evolving macro-economic assumptions as well as forecast uncertainty, the details of the macro-economic outlook and scenario assumptions as well as clarifications of provision variances over time, are set to be a particular area of focus.
Modeling Techniques in Scenario-Based Risk Appetite Management
To get senior stakeholders to buy in to alternative macroeconomic scenarios, risk management and ALM teams must assemble risk models and risk-adjusted performance measurements in their simulation tools. Institutions must switch from a qualitative to a quantitative approach to analysis.
Optimizing the Capital Ratio under Basel III
This paper explores the integration of credit and liquidity risk in Basel III, and shows how banks can optimize their capital under Basel III.