Efficient Asset Allocation with Least-Squares Monte Carlo
This article reviews the analysis of an asset optimization problem where risk is defined by the capital required under Solvency II principles, and where the portfolio performance is defined by the net asset value at time T=1.
Over the past decades, traditional asset allocation methodologies such as mean-variance optimization have been widely applied in the insurance industry. The benefits of such approaches for insurance portfolios have always been limited, due first to the complex interactions between assets and liabilities and second to the estimation difficulties and inappropriateness of asset portfolio returns and variances as target measures for portfolio optimization.
This paper analyzes an asset optimization problem where risk is defined by the capital required under Solvency II principles, and where the portfolio performance is defined by the net asset value (NAV) at time T=1. To overcome the technical challenges of relating the assessment of such measures in a market-consistent environment, the Least-Squares Monte Carlo (LSMC) approach as implemented in the B&H Proxy Generator was used.
The first section presents how firms can use LSMC techniques to generate allocation dependent proxy functions for the market-consistent value of asset and liabilities at both T=0 and T=1.
The second section builds on the concept to illustrate how firms can use these proxy functions to define an efficient asset allocation.
Presentation of the case study
By way of illustration, this article considers a book of with-profit insurance products with minimum guaranteed returns.
The initial asset allocation vector (sovereign bonds, corporate bonds, equity, property, etc.) is defined by Ainit= (50%, 20%, 20%, 10%).
The initial position of the balance sheet at T=0 is provided in Table 1.
Table 1. Balance sheet for allocation AINIT at T=0
Source: Moody's Analytics
The best estimate value of the liabilities has been calculated using 5,000 simulations generated from the B&H Economic Scenario Generator.
Four different classes define the investment universe:
- 10-year government bonds
- 7-year BBB corporate bonds
- The DJ Eurostoxx50 equity index
- Pan-European property index
In addition, it is assumed that the asset portfolio is rebalanced every year to the starting asset allocation.
A change in asset allocation will not impact the value of Assets at T=0, but will impact the value of the liabilities at T=0, and hence the NAV at T=0 through the impact on the cost of options and guarantees underlying the product.
A change in the initial asset allocation will also impact future balance sheet positions due to the following dynamics:
- Changes in returns on invested assets will impact the total value of assets and the value of the liabilities following the impact on allocated bonuses.
- Rebalancing rules applied to move to the initial asset allocation will introduce a secondary impact on liabilities.
Any change in asset allocation will require calculating the liabilities based on 5,000 trials. For many insurers, a single stochastic run for liability valuation will take several hours. As a consequence, insurers are usually constrained to limit their ALM analysis to a reduced number of allocations. Such ALM analyses are usually based on trial and error and can be time consuming. The next section shows how these computational challenges can be bypassed by using the Least-Squares Monte Carlo approach.
Asset allocation impact on T=0 balance sheet
This section demonstrates how the Least-Squares Monte Carlo technique can fit, with a high level of accuracy, proxy functions replicating the market-consistent value of liabilities and the net asset value (NAV) of an insurance portfolio for any combination of asset allocation, depending on proportions invested in different invested classes.
The proxy for the net asset value can be expressed as a polynomial function:
To perform the fit of the proxy functions, there are a total of 40,000 fitting scenarios used.
Next, the full stochastic ALM model for the following asset allocation choices is run in order to validate the convergence of the fitting functions (Table 2).
By comparing full ALM runs with the results provided by the proxy function, the quality of fit for both the NAV and market-consistent liabilities is seen.
The analysis in Figures 1 and 2 shows that even for very extreme variations in asset allocations, the fitted functions provide accurate estimations for both liabilities and the net asset value.
Once the function is validated, it provides an efficient tool to analyze the sensitivity of the net asset value to any changes in asset allocations, as shown in Figure 3.
Table 2. Asset allocation for the different validation scenarios
Source: Moody's Analytics
Figure 1. Out-of-sample validation for the proxy function of the NAV
Source: Moody's Analytics
Figure 2. Out-of-sample validation for the proxy function of the liabilities
Source: Moody's Analytics
Figure 3 shows that, as expected, allocation in assets with low volatility reduces the cost of options and guarantees, which has a positive impact on the net asset value. The interesting element here is that we can now obtain fast estimations of the NAV following changes in asset allocation.
Proxy fitting for T=1 balance sheet
The value of the liabilities at T=1 will depend on a larger number of parameters than the value of T=0; in addition to asset allocation, liabilities will depend on the market conditions realized at T=1.
This later component constitutes for many insurers the biggest challenge in the implementation of internal models, as the derivation of a probability distribution for net assets requires the execution of several thousand simulations.
To ensure that the LSMC technique converges with a high degree of accuracy, a number of mathematical conditions must be implemented with a high degree of precision. For the purpose of this case study, we used the B&H Proxy Functions Generator to generate both liability proxies and the validation results.
Figure 3.1. Weight of sovereign bonds when corporate bonds = 15% and property are = 10%
Source: Moody's Analytics
Figure 3.2. Weight of property when sovereign bonds = 20% and equity = 10%
Source: Moody's Analytics
Figure 3.3. Weight of equity when sovereign bonds = 5% and property = 10%
Source: Moody's Analytics
Figure 3.4. Weight of Sovereign bonds when corporate bonds = 10% and equity = 10%
Source: Moody's Analytics
The exposure to market conditions will be measured against the following six market risk factors:
- Two factors representing the risk-free yield curve. It is convenient to reduce the behavior of the yield curve to fewer variables. Here, the short rate and the mean reversion levels of the Libor Market Model are selected.
- An equity index stress
- A property index stress
- A factor representing the changes in volatility of equity
- One factor representing the level of corporate credit spreads
Additionally, the number of risk factors is extended in order to include the proportion invested in each of the asset classes – sovereign bonds, corporate bonds, equity, and property.
The net asset value is then defined as a polynomial function that can be written as follows:
There were 40,000 fitting scenarios used to generate function-fitting assets, liabilities, and NAV. Scenarios have been generated using the Stochastic Volatility Jump Diffusion (SVJD) equity model, LMM interest rate model, and G2 corporate credit spreads and transition model as implemented in the B&H Economic Scenario Generator.
Table 3. Asset allocation for the different validation scenarios
Source: Moody's Analytics
Figure 4. Out-of-sample validation for the proxy function of the NAV
Source: Moody's Analytics
Figure 5. Out-of-sample validation for the proxy function of the liability
Source: Moody's Analytics
In order to perform validations, the proxy function is compared to accurate valuations provided by the stochastic ALM engine for the following selection of parameters in Table 3.
Figures 4 and 5 show the validation results obtained at 15 different points, including extreme variations in market risk factors and asset allocations. For each of these points, the accurate estimation is based on 5,000 market-consistent simulations.
An accurate estimation of proxy functions offers two main benefits, the ability to:
- Perform projections of a market-consistent balance sheet and capital requirement under stress scenarios – part of the forward-looking capital assessment required under the ORSA component of Solvency II.
- Obtain a probability distribution for any balance sheet item at T=1.
This analysis has used the probability distribution of net assets in order to calculate the SCR of this book of business.
The calculation of the distribution is based on 100,000 real-world scenarios generated from the B&H Risk Scenario Generator software. To reduce the sampling error in the estimation of the 99.5th percentile to an acceptable level, using at least 100,000 trials is recommended.
The probability distribution of the net asset value can be an effective tool to derive an objective and robust risk appetite framework. It will also represent a powerful element in putting in place dynamic risk controls or to set risk-based performance targets within an organization.
Asset optimization
Traditional asset allocations aim to maximize asset returns and minimize the volatilities of the same assets. Due to the complexities in the calculations mentioned in previous sections, the analysis is usually restrained to the investment portfolio without considering the implications on the net asset value. As a consequence of these simplifications, the impact of variations in asset allocations on the starting value of the balance sheet is ignored in the optimization problem.
This section illustrates how to resolve the optimization problem when net asset value is defined as an indicator of an insurer’s wealth, and will analyze separately the cases where the expected value of the NAV and annual returns on NAV are chosen in the maximization objectives. The riskiness of the NAV will be defined by the SCR calculated from a probability distribution.
Figure 6. Distribution of the NAV of the initial allocation
Source: Moody's Analytics
Table 4. Stress testing focus: assessing shocks and how they transmit
Source: Moody's Analytics
The SCR at T=0 for a one-year forward capital assessment will be given by SCRt=1 = E[NAVt=1] – q99.5% [NAVt=1].
Figure 7 shows NAV versus SCR when running simulations for different combinations of asset allocation.
These results show that NAV is maximized and SCR is minimized when the allocation is done in low risky assets. This is not surprising from a shareholder’s point of view, as the impact of the risk on a market-consistent balance sheet is measured through the cost of options and guarantees. Depending on the relative size of the liabilities compared to the NAV, the cost of options and guarantees will dominate the expected risk premium on invested assets.
In the previous configuration, the optimization problem seems to be obvious; however, a with-profit insurer is also constrained when delivering minimum returns on assets in order to be competitive compared to cash investments or minimum rates offered by other insurers. This additional constraint requires the insurer to earn a minimum return on invested assets.
The colored circles in Figure 7 represent a different range of asset returns.
When we target a maximization of NAV, for instance, and if we assume that target investment return is 3%, then the optimal asset allocation is given by A = (25%, 15%, 50%, 10%), which corresponds to an expected NAV of 10,689 and SCR of 6,616.
An alternative approach to the optimization problem can be defined by maximizing returns on NAV instead of the absolute value of NAV.
Figure 8 shows the allocation diagram for returns on NAV versus SCR.
When looking at returns, instead of absolute value of NAV, the shape of the allocations have more similarities with the Markowitz efficient frontiers. The optimal portfolios are very different than the situation where absolute value of NAV is maximized, as optimal portfolios tend now to be those having the lowest starting NAV.
Table 5 shows optimal asset allocations under different optimization approaches, when the minimum investment return for policyholder bonuses is set at 3%.
Figure 7. NAV at T=1 / SCR for variation of two assets allocation
Source: Moody's Analytics
Table 5 shows that there can be significant variation in assets allocated depending on the measures considered for the risk/return couple. The choice of NAV vs. SCR provides, in this case, the highest NAV and the lowest SCR compared to other optimization approaches. An optimization based on return on investments vs. SCR provides the worst portfolio with the lowest value of NAV and the highest SCR.
Table 6 shows optimal asset allocations under different optimization approaches when the minimum investment return for policyholder bonuses is set at 2.5%.
A reduction in bonus rates lead to an increase in the proportion invested in government bonds given that a lower risk premium is required. Again, the optimization based on NAV vs. SCR provides the best allocation with this highest NAV and the lowest SCR.
An asset optimization focusing only on the distributional characteristics of an investment portfolio will often not lead to an optimal portfolio from the perspective of value creation for a life insurance firm. The use of simplifying assumptions that ignore asset and liability interactions is often driven by computational challenges in the valuation of the insurer’s market-consistent balance sheet.
This article extended the use of LSMC technique beyond its usual application to the SCR calculation. The same single functional form can be used to maximize the net assets of an insurance firm for a wide range of asset allocations. The flexibility of the functional forms gives the ability to integrate a large number of constraints, such as regulatory/economic capital or targeted minimal bonuses for policyholders.
The internal models have become an effective decision-making tool, allowing management to assess the appropriateness of each strategic action under the numerous interdependent criteria that exist within an insurance company. The ability to embed the internal model within the decision process also provides a good proof on the use test criteria required under Solvency II for the validation of internal models.
Figure 8. Return on NAV / SCR for variation of two assets allocation
Source: Moody's Analytics
Table 5. Optimal asset allocation when minimum expected return on invested asset of 3% for policyholder bonuses
Source: Moody's Analytics
Table 6. Optimal asset allocation when minimum expected return on invested asset of 2.5% for policyholder bonuses
Source: Moody's Analytics
Notes
1 Multiplicative shock on the indices.
2 Multiplicative shock on the level of short rates and mean reversion.
3 Shock on the starting value of the spread CIR process.
Featured Experts
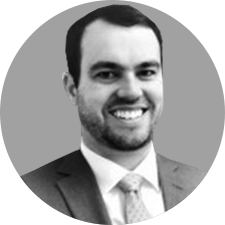
Scott Dietz
Scott is a Director in the Regulatory and Accounting Solutions team responsible for providing accounting expertise across solutions, products, and services offered by Moody’s Analytics in the US. He has over 15 years of experience leading auditing, consulting and accounting policy initiatives for financial institutions.
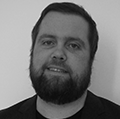
Paul McCarney
Insurance product strategist; insurance domain expert; extensive experience developing risk assessment frameworks for insurers
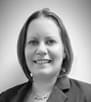
Karen Moss
Senior practitioner in asset and liability management (ALM) and liquidity risk who assists banking clients in advancing their treasury and balance sheet management objectives
As Published In:
Addresses the challenges and opportunities in the global insurance sector, and how they impact the risk management practices of insurers.
Previous Article
Arbitrage-Free Scenarios for Solvency IINext Article
Spotlight on Suncorp GroupRelated Articles
The Next Wave – Implementing a well-designed Internal Model
Institutions are transforming their analytic capabilities to move beyond static reports that explain what happened in the past, to more modern analytics that can explain why an event occurred and what is likely to happen in the future.
Efficient Asset Allocation with Least Squares Monte Carlo
Asset optimization which focuses only on the distributional characteristics of an investment portfolio will fail to achieve an optimal portfolio from the perspective of value creation for a life insurance firm. In this paper we show how this issue can be resolved through the application of Least Squares Monte Carlo techniques.